Sep 2, 2015 · post
D’Alembert’s Deep Dream: Bees and Nonlinear Transformations
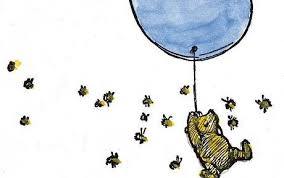
Hold your hats! In the next couple of weeks we’re launching an arsenal of deep learning resources, including a feature report, a public prototype that will classify your Instagram identity and a webinar exploring the past, present and future of deep learning. Sign up here!
As a philosophical prelude to the upcoming report, we wanted to invite you to think about the emergent properties of neural nets. Let’s explore what 18th-century philosopher Denis Diderot can teach us about artificial intelligence.
Diderot was not your average Enlightenment philosopher. A philosophe, he grappled with the colossal inheritance of mechanical philosophy that dominated 18th-century intellectual circles. Spearheaded by Descartes and Newton, the mechanical view held that the material world was composed of complicated machines governed and determined by immutable laws.
But Diderot and a few contemporaries noticed that Newtonian mechanics, while powerful for describing celestial phenomena, did a poor job describing living organisms. Arguing against his predecessors, Diderot proposed that life - like sentience and consciousness - emerges from the complex interaction between many small constituent parts. He illustrates the idea in his 1769 “D’Alembert’s Dream” with the metaphor of a swarm of bees:
“Have you sometimes seen a swarm of bees going out of their hive? This cluster is like an individual. If one of the bees pinches the bee next to it, the second bee would then pinch the one next to it in turn, until in the entire cluster there would be as many sensations aroused as there are small animals. Someone who had never seen a group like that arrange itself would be tempted to assume it was one single animal with five or six hundred heads and a thousand or twelve hundred wings…”
Anyone familiar with Google’s Deep Dream might picture D’Alembert’s twelve-hundred winged swarm of bees like the many-eyed mutants generated by Google’s API. When I imported an image of a beehive, Deep Dream transformed it into a two-headed duck-rooster placidly perching in a tree:
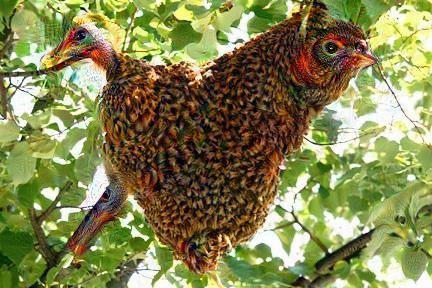
But Google did not create Deep Dream only to generate psychedelic images. Rather, Deep Dream is a tool to investigate how neural networks identify features in images and encode these features at each of their various layers.
Indeed, one of the drawbacks of convolutional neural networks underlying image-object recognition work is that the very feedback mechanisms that enable us to train the network generate an “unpredictable” system that, according to Slate columnist David Auerbach, is neither rational nor algorithmic.
Convolutional networks use a class of algorithms called “kernels” that encode the spatial features of images (e.g. the sharp edge of a beehive has the same features of a duck beak) into matrices; neural nets then use vectors and linear algebra to transform the data layer by layer, first to encode the data’s features and then to filter them based on how a model has “learned” to categorize those features. But as these transformations are nonlinear, we cannot identify what features each layer is encoding.
As such, the behavior of the neural network emerges from the complex interaction at play between the various layers. To return to Diderot, we might envision the various nodes in a neural network like individual bees that, when swarming together, generate a whole that supersedes the sum of its parts to become something new.
Will these emergent properties, then, be the secret to generating artificial sentience and consciousness? John Stuart Mill, after all, claimed that the human mind emerged from the complex interaction of brain matter. But, as we explain in our upcoming deep learning report, neural networks are extraordinarily rigid in contrast to the plasticity of the human brain. To learn more, join us for the webinar we are hosting September 17!
-Kathryn