Oct 6, 2015 · post
Breaking Down Memes
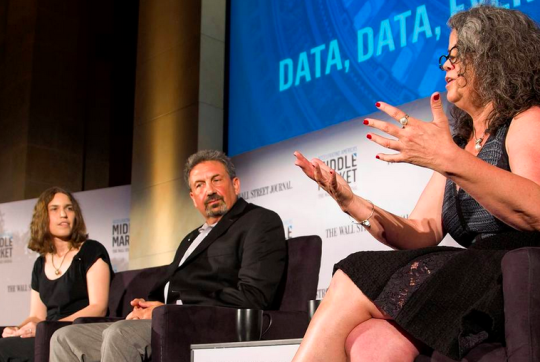
Last week, Hilary spoke about opportunities for mid-sized companies to use data at Accelerating America’s Middle Market, hosted by the Wall Street Journal. This morning, I spoke about opportunities for large, established companies to use data at a Corporate Longevity Leadership Briefing, hosted by the Financial Times. Here are two key takeaways from the panels:
Memes generate excitement; excitement generates hype; hype generates confusion. In her panel, Hilary aimed to demystify some of the sorcery surrounding ‘big data’ that risks rendering data projects intimidating to mid-sized companies with limited resources. “This is all extremely common-sense stuff,” she said. “It’s as simple as collecting information, counting something in it and maybe averaging it or normalizing it in some way and getting an answer out and maybe making a graph of that answer.” Still, a healthy dose of skeptical humility is hallmark trait of a good data scientist. We often speak with executives of mid-sized private companies who have already told themselves the story they want their data to tell. But the real excitement in data lies in its forcing executives to step back and think critically about the assumptions underlying their strategy. There’s a few basic questions that work for most projects: “What question about our business do we want to verify using data? Can our existing data help answer that question? If not, what data do we need to collect to answer the question in a meaningful way?” Often the smartest insights arise when we have the courage to think about the basics.
Another meme that merits demystification is ‘disruption innovation.’ In his classic The Innovator’s Dilemma, Clayton Christensen classified technology innovations into two categories: sustaining and disruptive. Most tech advances are sustaining, helping companies continue to do what they’ve always done, just faster and with features increasingly tailored to business processes. Some advances are disruptive, truly reshaping how markets perform a certain activity. Per Christensen, it’s because technology advances more quickly than market demands that ‘disruptive’ startups can unseat the large companies that excel at sustaining innovations. But the world of data and machine learning muddies the waters of Christensen’s innovation theory. Machine learning algorithms must be trained using large data sets to function optimally. Large, established companies have lots of data. Disruptive startups don’t. We believe this generates novel opportunities for collaboration between startups and large companies, where innovation depends upon the symbiosis between researchers and enterprises. At Fast Forward Labs, we enable enterprises to start preparing for the technology trends that will undoubtedly shape their future as they help us understand the potential of new algorithms in greater depth by testing them in practice.
Moral of the story? Big data gets smaller if you stick to the basics. Innovation is a vehicle for longevity when the startups and corporations partner to shape the future.
-Kathryn