Sep 29, 2015 · post
Machine Learning in Retail: Consumer Privacy Implications
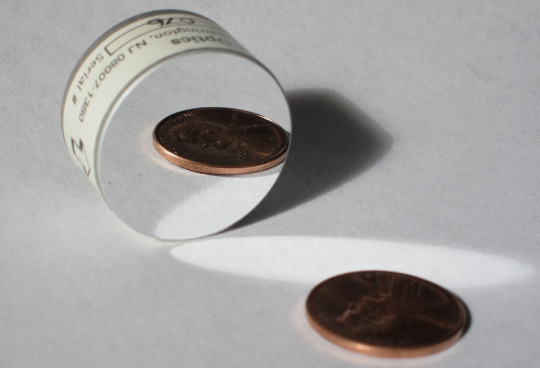
In our last retail post, we explained how emerging sensor technologies are changing data science for brick and mortar. Many companies are working to retro-fit physical stores with capabilities originally developed for ecommerce. Without adding any new sensors or tags, image recognition company Blippar provides APIs that digitize physical products to create an in-app experience around them. Farfetch, which initially brought an exclusive network of luxury brick & mortar shops online, recently announced that it will soon provide in-store analytics.
In parallel, digital companies continue to seek out physical spaces to augment their customer experience. Warby Parker and Rent the Runway have physical stores, and Gilt and Etsy are exploring new models to engage consumers offline (Gilt has a private retail space in their corporate headquarters and Etsy launched an app to inform shoppers of sellers’ nearby products). These digital companies were built upon testing every detail affecting their website performance. As tracking migrates to physical retail, it’s important that retailers consider the consumer experience risks associated with new technologies as they explore the benefits.
“When you look at something on the web, you get ads that pop up and follow you around - companies like that have much better advantage over brick-and-mortar retailers, and so they’re under more pressure to equalise the playing field.” Kevin Kearns, ShopperTrak
Reasonable Expectations for Privacy
Before retailers invest resources to model consumer data, they should ask whether users would respond better to less personalized advertising experiences. There’s a difference between personal preferences and personal traits. A personalized experience that tends to an individual’s preferences can feel bespoke, high-service, and attentive to the consumer’s needs. A personalized experience generated by traits or demographics can feel more like differential treatment, where the experience is triggered by a collection of “contexts” sensed about the consumer.
Adobe and Page Fair point out that there are nearly 200 million active users of ad blocking software, costing advertisers about $22 billion dollars a year, with 48% growth in the US over last year. In addition, in a 2000-person survey from Altimeter Group, all age groups expressed concern about data collected in public, with specific discomfort around use of body data. Retailers should keep consumer tolerance in mind as new sensors and software makes it easier to capture extremely detailed profiles of in-store shoppers.
Technology always outpaces policy and law, and to date retailers lack precedent for providing consumers with privacy policies articulating passive data collection practices. Some tech companies, like Prism Skylabs sidestep privacy concerns by anonymizing personally identifiable data, giving “a sense of what’s happening in a store without having to be there physically counting customers or examining shelves.” Neiman Marcus, alternatively, asks for explicit consent to record gender, age, and body type data when testing their Memomi smart mirrors.
After a decade of digital ecommerce, many people’s expectations regarding online privacy have shifted to expect monitoring by retailers and advertisers. But the same does not hold in physical retail, where past methods of sales tracking amounted to conversations with sales associates or, at most, wifi tracking using mobile phones. But emerging technologies can do far more than track location: they can add sophisticated “metadata” about a consumer’s age, body measurements, and browsing habits.
To think through privacy expectations, researchers Soultani and Bankston developed a model that relates individual expectations of privacy to technology costs. “If the cost of surveillance using the new technique is an order of magnitude (ten times) less than the cost of the surveillance without using the new technique,” they claim, “the new technique violates a reasonable expectation of privacy.” So what are the economics of the new tracking tools? Nomi’s Brickstream sensor costs as low as $20/month for a plan of 25 in-store sensors, amounting to at least $500/month (pricing may vary over time). If tracking consumer preferences - or bodies - were left to a sales associate making $28K/year, monthly costs would amount to at least $2333, notwithstanding the fact that a single person’s attention cannot scale across the customer base. The cost to track a single person quickly falls near $0 for a sensor that scales across the customer base. If we trust Soultani and Bankston’s model, the prices of these new tools trigger a re-evaluation of best practices for policy around privacy.
Technology Limitations Beyond Privacy
Even if consumers tolerate passive data collection, algorithmic pitfalls also impact user experience. Many machine learning systems learn using training datasets, which impact the predictions they make about new data in the wild. As boyd and Crawford point out, “a data set may have millions of pieces of data, but this does not mean it’s random or representative.” Imagine generating a body-specific ad, or even matching existing inventory to a consumer’s body measurements. What happens if there is not enough data to generate a realistic representation of an individual? If nothing in a store’s inventory fits a customer’s body? If a 15-year-old girl’s body best matches 40-year-old women in your database? Would automated suggestions pull up inventory more appropriate for older customers, alienating her in front of friends with bodies more like the average 15-year-old?
A careful, opt-in experience does have the power to create experiences that were much harder to scale in the past. Companies like Slyce, Cortexica, Deepomatic, and Donde Fashion are harnessing deep learning to augment the browsing experience. During New York Fashion Week this month, Cortexica pointed out the challenge of finding the right item when a shopper brings a photo of what he/she wants that might not exactly match inventory. Without requiring an item tag, their software analyzes photo data to suggest items with similar colors and textures, within or across detected categories. Here, software augments customer preferences rather than prescribing preferences from passive data collection about multiple consumers. Shopping is all the more fun when a photo of a sunflower is game for inspiration like a street style star.
-Jessica