Nov 17, 2015 · post
When Dog Is Enough: Using Hypernyms To Improve Neural Network Predictions
Possibly true statement: the Fast Forward Labs dog is the cutest dog in the world.
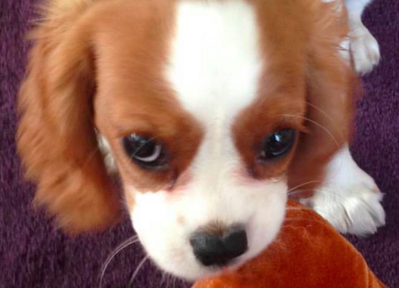
Our General Counsel Ryan picked up the puppy a month ago and we’ve yet to name him. Ryan likes Renfield, which, as Bram Stoker fans know, evokes slightly different thoughts than “super cute,” particularly when played by the ever-guttural Tom Waits. But the fact that we’re in no rush to name him tells us something about how we label and identify things. We know he’s a dog, we love him for his dogness, and thus far that’s been just fine. I personally tend to forget what breed he is, as my knowledge of dog breeds is shamefully sparse.
Pictograph, in contrast, does an excellent job recognizing that our puppy is in fact a blenheim spaniel. Pictograph is the public app we built to illustrate how neural nets identify objects in images. Try it on your personal Instagram feed!
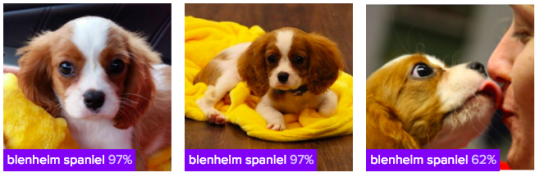
A 97% confidence rate in the accuracy of the prediction is a dream for automated classification. Here, the confidence is so high for two reasons.
First, the ImageNet database used to train the Pictograph neural network has a lot of pictures of blenheim spaniels (971…and yep, it’s prime). This labelled data informs the network what a correct classification should look like. The learning mechanism (called backpropagation) then steps in and learns the network to predict the label “blenheim spaniel” when presented with new images that have similar features.
Second, the images in Ryan’s Instagram feed aren’t noisy. Note how the two images with 97% confidence rates show our puppy alone and facing the camera. This pose is similar to the stock images available on ImageNet, rendering it easier for the neural net to detect similarities. The confidence rate in the right-hand image including the human face drops to 62% because the data is noisier. It would likely drop further when presented an image of our puppy unconsciously playing Ouroboros (the mythical snake that eats its own tail).
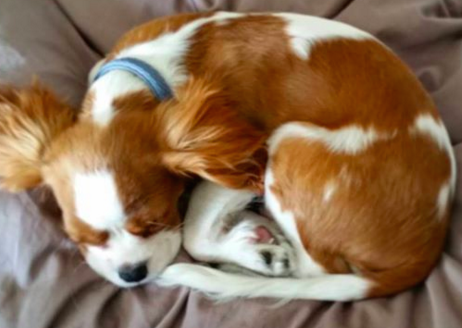
But Instagram, like most data in the wild, rarely contains clean data that maps neatly to a model’s parameters or the stock photos in a training set like ImageNet. In turn, classification systems can yield confidence rates as low as 20% or 30% (or lower), generating doubts as to whether it’s worth using the technology at all. One way to improve unsatisfying results from a machine learning tool is to adopt a “human in the loop” approach, where humans step in and manually label images technology misclassifies or classifies with low confidence rates. But we decided to adopt a different approach.
ImageNet and WordNet
ImageNet is not just a collection of images arranged arbitrarily. It is organized according to the hierarchy found in the lexical database WordNet, whose “structure makes it a useful tool for computational linguistics and natural language processing.” Like a thesaurus, WordNet groups different nouns (we’ll focus on nouns because ImageNet does not use verbs, adjectives, or adverbs) together into sets of synonyms, or synsets. Synsets express concepts (e.g., car = automobile) between which WordNet can generate relationships.
In WordNet, the most frequent relation among synsets is hyponymy and hypernymy. A bit of Ancient Greek helps here: onomas means name; hypo means under; and hyper means over. So a hyponym is an “undername,” a more specific instance of a concept (blenheim spaniel is a hyponym of dog). And a hypernym is an “overname,” a more general instance of a concept (domestic animal is a hypernym of dog). In WordNet, all noun hierarchies ultimately go up to “entity.” (A bit more Ancient Greek has muddied the metaphysical waters for centuries, with Aristotle’s unmoved mover inspiring Spinoza’s causa sui, but I digress…)
Note that a hyponymy relation is transitive: if an blenheim spaniel is a kind of dog, and if a dog is a kind of domestic animal, then a blenheim spaniel is a kind of domestic animal. And here is the key: a neural network can use this transitive property to manage low confidence predictions. How?
Using Clustering to Unlock Network Intelligence
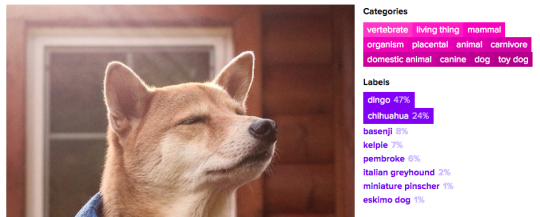
Consider this image. Fathom, the advanced deep learning prototype we provide to our clients, isn’t sure whether this is a dingo (47%) or a chihuahua (24%). One approach would be to simply select the maximum likely prediction and label this a dingo (which I believe is correct?). But that ignores other information the network is providing. A 24% confidence rate that this is a chihuahua, a 8% confidence rate that this is a basenji, and no mention that this is a siamese cat or an Arabian stallion, all tell us something: that this is not a cat and not a horse, but some type of dog. Even if the network does not deliver the most precise knowledge possible with absolute confidence, it can use the transitive relationship between hypernyms to know this is a dog (and a domestic animal, and, well, an entity of some sort).
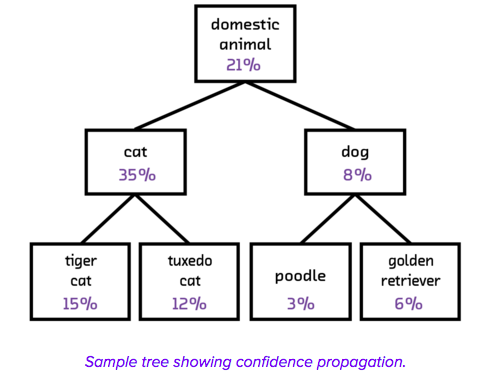
Augmenting label predictions through a clustering scheme can be incredibly important to make neural networks valuable in practice. Data teams constantly face challenges in managing noise, and those challenges won’t disappear with deep learning. The quality of the results will always depend on the extent to which new data is like or dislike data in the original training set; this relationship is further impacted by the size of the training set.
When considering a deep learning project, one initial question data teams can ask is how important it is for the given problem that the neural net return extremely precise classifications. If you’re using a neural network to identify tumors in MRIs, you may need to go for maximum likelihood. But if you’re using the tools for fashion retail, knowing that something is a skirt as opposed to a Marc Jacobs skirt may be good enough (happy to be corrected).
After all, we may have an anonymous office dog, but that doesn’t mean we don’t adore him.
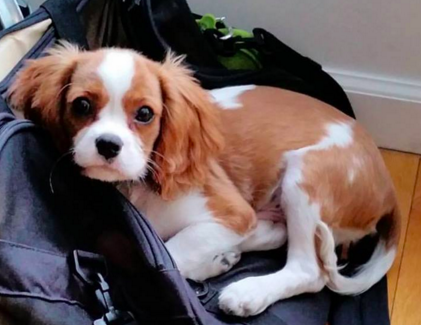
-Kathryn