Feb 18, 2016 · interview
NeuralTalk with Kyle McDonald
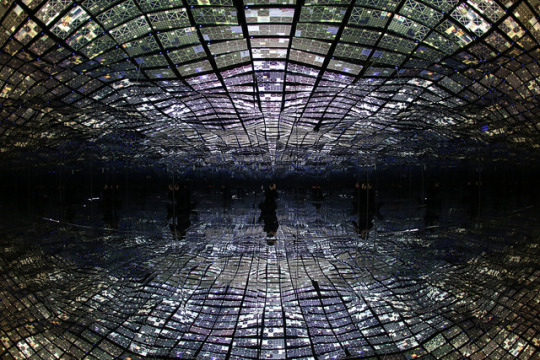
Image from Social Soul, an immersive experience of being inside a social media stream, by Lauren McCarthy and Kyle McDonald
A few weeks ago, theCUBE stopped by the Fast Forward Labs offices to interview us about our approach to innovation. In the interview, we highlighted that artists have an important role to play in shaping the future of machine intelligence. Unconstrained by market demands and product management requirements, artists are free to probe the potential of new technologies. And by optimizing for intuitive power or emotional resonance over theoretical accuracy or usability, they open channels to understand how machine intelligence is always, at its essence, a study of our own humanity.
One provocative artist exploring the creative potential of new machine learning tools is Kyle McDonald. McDonald has seized the deep learning moment, undertaking projects that use neural networks to document a stroll down the Amsterdam canals, recreate images in the style of famous painters, or challenge our awareness of what we hold to be reality.
We interviewed Kyle to understand how he understands his work. Keep reading for highlights:
How did you become an artist using machine learning? Did you start as a technologist and evolve into an artist, or vice versa?
I started as a curious person, and this manifested itself in multiple ways. I started exploring the intersection of algorithms and music at the end of high school with a lot of Perl, ActionScript and QBasic. Going into college I knew I wanted to do something with machine intelligence, to develop generative systems that could mirror human creativity. But as it turns out, machine intelligence research rarely focuses on creativity, but solves relatively mundane problems. Top researchers at the time were detecting fraud or recognizing handwriting on checks, not creating artwork. So I changed focus, moving to new kinds of musical interfaces, and eventually interactive installations. Over the last year I’ve returned to machine learning because it seems like there is a renewed interest in machine creativity. People are asking deep questions rather than just solving problems or improving accuracy and performance.
Eyeshine captures, records and replays the red-eye effects from the eyes of its observers. Collaboration with Golan Levin.
What’s changed over the past year to make machine learning interesting again for artists?
Sometimes research efforts designed to serve a given purpose serendipitously become catalysts for art and creativity. Take deep neural networks. Often, the only academic justification for playing with the generative capabilities of models is to improve understanding of the data or to provide new data to help train other algorithms (e.g., to improve training on a support vector machine). Google even designed Deep Dream to better understand how neural networks process images. But when we, as human observers, encounter the output of this research tool, we interpret this as a glimpse into the imagination of the computer. This, as well as techniques like style transfer, open up the floodgates for creativity. Both for people working with the techniques, but also for those inspired by the narrative of the tools.
What is it about neural networks that render them particularly apt for creativity and art?
One particular benefit of neural networks is how easy they are to mentally manipulate. Once you understand a basic artificial neural network and how it works, you can intuitively progress to autoencoders, which help with feature extraction and dimensionality reduction, or to recurrent neural networks, which do well with sequential data of variable input length, like text. Given this adaptability and generalizability, creative people can reframe different types of tasks in terms of neural networks, as opposed to pigeonholing everything as, say, a classification or regression task.
What are you after when you use deep learning for artistic purposes? Are you exploring machine creativity or human creativity?
I’m interested in computational systems because they reveal something about the designers. Studying machine intelligence could be seen as a kind of philosophy, psychology, or even anthropology: a discipline built to probe the structure and rationale behind human existence and interaction. I don’t just mean that machine learning enables us to tease insights from big sets of data that depict humanity as an abstraction. I mean that in building these models, and observing their output, we learn something about ourselves. As an artist, I’m not after the most accurate model, but the model that can give us a stronger intuition and understanding of what it means to be human, that can help us change our perspective, that can make us feel something we’ve never felt before.
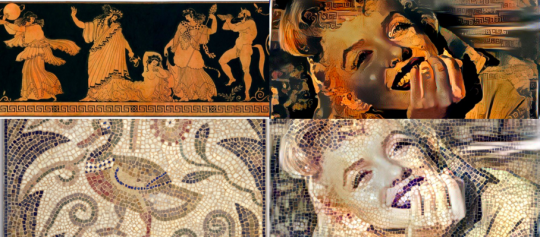
McDonald grafts art history onto a photo of Marilyn Monroe
Can you give an example of something we can learn about ourselves from AI?
The current discourse claiming that AI poses existential threats to humanity reminds us of the human tendency to fear the other. The discussion is framed in moral, normative terms: is AI good or bad? Will it get better or worse? Political overtones seep in even though we’re describing not humans or human agency, but an abstract potential that is hard to grasp and understand. There’s also our discomfort towards the so-called “uncanny valley,” the emotional response we feel when we encounter an entity that is almost, but not quite, human. There’s lessons here that could help us reflect on how we treat other humans.
What are some examples of uncanny machine intelligence?
After DeepMind released the Nature paper about using neural networks and tree search to master Go, a Korean Go player remarked they must have used a database of Japanese players because the system exhibited a Japanese playing style. Champions infer their understanding of how a human player would play onto the machine, just like Kasparov was surprised when Deep Blue made a move that didn’t feel like algorithmic chess. It’s analogous with text generated from Long Short-Term Memory networks, which help recurrent neural networks keep track of long-term dependencies in sequential data. The algorithms sometimes generate surprising, strange words that we impose meaning on, turning them into poetry.
Your work seems to fall into two buckets. Some of it clearly seeks to defamiliarize the viewer, like the Augmented Hand Series that “prompts a heightened awareness of our own bodies.” The other seems to be more passive and documentary, like Exhausting a Crowd. What is “Exhausting a Crowd” about?
This piece is inspired by “An Attempt at Exhausting a Place in Paris,” an experimental novel Georges Perec wrote from a bench over three days in 1974. Perec effectively shares his subjective experience with others, giving others his personhood by enabling them to see through his eyes. In today’s digital world, our concept of individuality is expanding because we can shift between personae and personalities by clicking across accounts. The boundary between the self and the collective has always been blurry, but now we can see just how blurry it is. I wanted to revisit Perec’s project, but orient the perspective towards a collective vision that included both humans and machines. To that end, “Exhausting a Crowd” automates the task of completely describing the events of 12 hours in a busy public space. I began the work using neural-talk image captioning, but ended up using only human-generated tags and labels to emphasize the feeling of surveillance.
McDonald uses Andrej Karpathy’s “NeuralTalk” code on a webcam feed
Georges Perec was an early member in the French algorithmic literary group Oulipo. What other artistic movements inspire your work?
Oulipo and Dada are huge influences when it comes to understanding the role of the artist in culture. The Situationists and Fluxus frame everything else, from performance to interaction. I’m intrigued by these older movements that aim for conceptual and not merely aesthetic value.
When you use algorithms to generate art, who’s the artist: you or the machine?
This question holds for all artists, whether they work with machines or not. We all have a multitude of influences from culture and individuals both recent and ancient. And it doesn’t stop with the artist: the observers and participants who join in appreciating the work continue to recreate it. Our agency is diffuse and collective.
You mention on your website that you spend a significant amount of time building tools for other artists. Where do you see yourself in the scientific and artistic communities?
Sometimes I feel like I’ve stumbled into the river between the arts and sciences, so I offer tools as a bridge to help people who want to cross but don’t have the same opportunity to go swimming. Working at the threshold of machine learning and art is like working on perspective in the Renaissance, where there was a fruitful collaboration between scientific and artistic modes of thinking. As an example, deep learning researchers are all working with huge batches of data to train their networks. But from my experience working on interactive installations I know you learn the most when something is happening in real time. Rebecca Fiebrink at Goldsmiths has been doing this for a while with Wekinator, and is getting great results.
What are you working on next?
With all the emphasis on text and images, I’m trying to focus on sound and music. I’d like to hear nets generate new compositions, or create “style transfers” of existing recordings. There is some good composition work, from Doug Eck’s LSTM blues to Bob Sturm’s generated folk music, or Daniel Johnson’s classical piano compositions. But everything still sounds, at best, like David Cope’s experiments in musical intelligence, which was a finely-tuned but much simpler algorithm.
Instead of thinking of music as a sequence of symbols that can be embedded and mapped to vectors like text, I’m curious to see what happens with raw audio content. One challenge in working with music is that the structure of the music happens at a different scale than the structure of the sound. Working with raw audio is like trying to learn to spell words, and then jumping straight to writing a novel. But it’s a challenge I’m excited to embrace.