Feb 16, 2016 · guest post
Machines and Metaphors

This is a guest post by Gene Kogan, an artist and programmer who applies emerging technology into artistic and expressive contexts, and teaches courses and workshops on topics related to code and art.
Recent advances in deep learning research have renewed popular interest in machine intelligence. With new benchmarks set in tough problems (e.g., image classification and speech recognition), researchers are exploring unexpected and exciting applications, and eliciting public engagement and private investment. These recent breakthroughs have captured the attention of many for whom AI was previously obscure, as new capabilities spur applications of interest to wider public audiences.
But these advances have captured more than just our attention; they’ve captured our imagination. Artists have been quick to apply these new techniques for novel creations, exploring the uncharted territories of machine creativity, slyly provoking questions of greater importance. What is creativity anyway? How do machines perceive, learn, and imitate?
When an algorithm is taught to paint the Mona Lisa in the style of van Gogh’s Starry Night, it doesn’t just demonstrate an ability to paint like van Gogh; it demonstrates a much more general ability to emulate human behavior. Taken further, it shows the capacity of an algorithm to take bits of seemingly disorganized and meaningless morsels of data–pixels, characters–and abstract knowledge from them which is quite meaningful to humans. Even the world’s foremost researchers are admittedly awestruck by these results. We may understand the math, but the intuition for why it works escapes us.
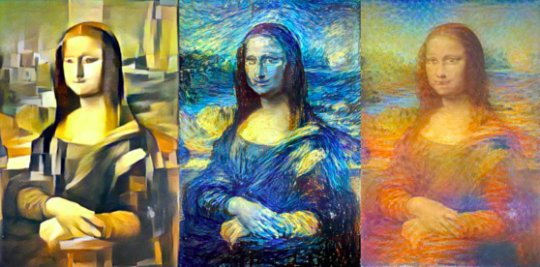
neural-style of Mona Lisa in cubist, expressionist, and impressionist form
Artistic applications can help mitigate this uncertainty, and naturally, many are initiated within the research community itself, sometimes with intentionally creative undertones. Deepdream and style transfer (or “neural style”, “#stylenet”) were publicly released by researchers, piquing the curiosity of many practitioners online (including myself) who riffed on the software to create countless artworks. Both received a great deal of mainstream press coverage [1][2][3][4][5][6], becoming among the first examples of machine-learned generative art being shown to wider audiences. Using Justin Johnson’s popular neural-style implementation, I produced a restyled version of a scene from Alice in Wonderland. At the time, the results seemed too good to be real, but I had a hunch that more clarity would arrive downstream.
The implementation of a deep convolutional generative adversarial network (DCGAN) published on Github by Alec Radford, Soumith Chintala, and Luke Metz is a good example of research partially motivated by creative goals. Describing their algorithm as “tripping” and packing the README with troves of machine-hallucinated psychedelia, they seemed to be deliberately inviting artists to repurpose their code. So I took the bait and trained a DCGAN to generate animated interpolations of handwritten Chinese characters. Others applied the technique to produce fake flowers and manga characters, eliciting praise from Yann LeCun and others.

excerpt from A Book from the Sky
Spurred by these and others, more creative machine learning hacks have appeared in recent months, including another project involving Chinese characters by @hardmaru. Here, the artist invented wholly new characters from a trained recurrent neural network. Continuing with the theme of written language aesthetics, Erik Bernhardsson explored the latent space of typography, training a network on 50,000 fonts. Another strain of research, combining convolutional and recurrent neural networks, has produced software capable of annotating or describing images with natural language [1][2], and even more experimentally, the reverse. These hint toward a future in which machines can autonomously process information multi-modally, exchanging text for images or sounds, and vice-versa. These implementations reflect a growing interest of those with more technical backgrounds to apply their research in artistic and design-focused ways.
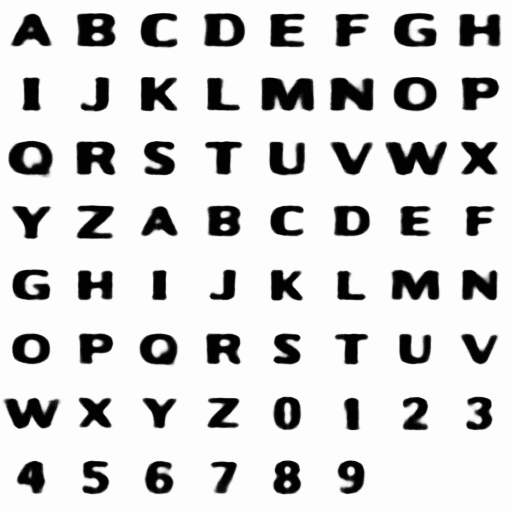
excerpt from Erik Bernhardsson’s font-generating neural net
Conversely, those coming from artistic backgrounds have been delving deeper into scientific literature, reading public papers on arxiv.org, and designing their own deep neural networks using open-source frameworks like Caffe, Theano, Torch, and TensorFlow, which have dramatically eased the process of getting started. Even higher-level libraries on top of these frameworks have appeared, including Keras, Lasagne, Blocks, and others. The tools of the trade for artists and scientists alike have been converging, blurring the distinctions between them and facilitating new lines of inquiry and cross-disciplinary dialogue.
The rise of these powerful tools has precipitated an explosion in public discourse about machine learning. The ML subreddit, once a treasure chest of wild speculation, is now rife with students and amateurs asking technical questions and sharing project ideas. Many of the authors of the libraries mentioned above actively maintain blogs where they discuss the latest discoveries, often sharing code for how to reproduce them.
One researcher, Andrej Karpathy of Stanford and OpenAI, even released a Javascript implementation of a convolutional neural network, convnet.js. One may wonder why, since Javascript’s limitations give this library little applicability to scientific research. But that isn’t the point. Embedded in a web page, the neural network is instantly accessible: anyone with an internet connection can now interact with an algorithm that has revolutionized computer vision and speech processing.
As these new methods improve and mature, they will rapidly be applied in contexts that could have significant consequences for society, raising the stakes for their use. This creates more urgency for outreach and education, to inform the general public about these multifaceted and counterintuitive technologies. As exciting as they are, they also risk misuse. The example usually cited is how a self-driving car would choose which of two pedestrians to kill if it could only avoid one. Although this scenario is a bit exaggerated, it’s an example of a decision a machine would inevitably have to make. As implementation decisions along the way introduce biases and affect the outcome, the public should have a say in influencing their development.
Fortunately, the deep learning research community largely conducts research openly, embracing open-review platforms like arxiv and publishing open source software. Additionally, libraries wrapping machine learning functionality into creative coding toolkits like openFrameworks provide an avenue for artists to probe deeper.

images of animals clustered in 2D using openframeworks libraries ofxCcv and ofxTSNE
But the fact that tools are open source does not mean the public understands what those tools entail. Along the journey from initial scientific research and to mass deployment of machine intelligence, artists can help illuminate the gap, providing accessible and engaging cultural metaphors which are more readily understandable than the layers of abstraction in pure research. The machine metaphor has been successful in the past, helping to popularize computer vision in the context of interactive installations, celebrating its playful side while simultaneously raising caution about its lesser known properties. If present trends continue into the near future, machine intelligence could follow a similar path.
- Gene Kogan