Apr 11, 2016 · announcement
New Tools to Summarize Text
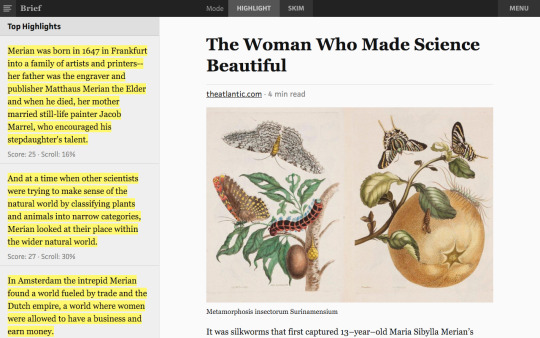
We’re excited to introduce the latest report and prototype from our machine intelligence R&D group! In this iteration, we explore summarization, or neural network techniques for making unstructured text data computable.
Making language computable has been a goal of computer science research for decades. Historically, it has been a challenge to merely collect and store data. But it’s now so cheap to store data that we often have the opposite problem: once we’ve data, how should we analyze it to find meaning and insights?
Many organizations have made good headway processing structured, transactional data for Business Intelligence, but few have extended analytics to compress insights from the emails, news articles, reports, legal documents, and other troves of written documents that make up the lifeblood of organizations.
But we’re beginning to gain the ability to do remarkable things with unstructured text. Businesses that adopt this technology will see significant advantages. They will find important information faster. They will expand the horizons of how and what they read, gleaning actionable insights from document corpuses too large for humans to process.
Our work addresses multi- and single-document summarization, illustrating the best technical approaches with two prototypes. Our multi-document prototype uses Latent Dirichlet Allocation to map topics and collect key points of view across thousands of Amazon product reviews.
Our single-document prototype, Brief, uses skip-thoughts and recurrent neural networks to extract the sentences that best represent the key ideas in a longer document. You can see how Brief scores and highlights an article’s most interesting sentences in our public preview.
Our report records lessons we learned building our prototype, teaching readers:
- how different algorithms represent unstructured text quantitatively
- why recent breakthroughs in deep learning allow us to model meaning
- how to build a summarization system and setbacks to avoid
- who the key summarization vendors are and what they offer
- where natural language processing will go in the near future
We’re excited to help our clients identify opportunities to use these capabilities in their businesses, be that to facilitate research on investments, find documents relevant for a legal matter, manage email overload after vacation, or automatically generate tweets. What else do you imagine?
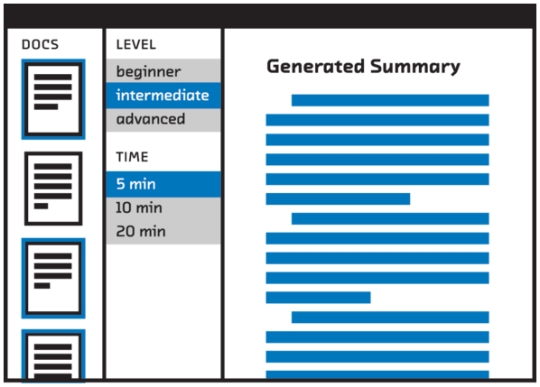
Please [contact us](mailto:cffl@cloudera.com) if you’d like to learn more about our research or advising services.
And join us May 24 for an online discussion with New York NLP startup Agolo, where we’ll explain how summarization technology works and how businesses are using it today!