Mar 30, 2016 · interview
Shivon Zilis on the Machine Intelligence Landscape
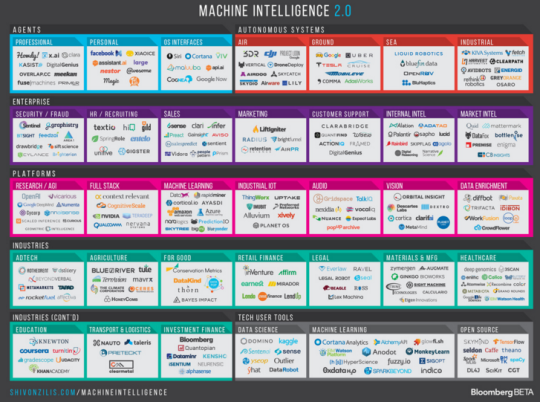
2016 is shaping up to be a big year for machine intelligence. Achievements like DeepMind’s AlphaGo are making headlines in the popular press, large tech companies have started a “platform war” to become the go-to company for A.I., and entrepreneurs are increasingly building machine learning products that have the potential to transform how companies operate.
Exciting though the hype may be, the commercial potential of machine intelligence won’t be realized unless entrepreneurs and data scientists can clearly communicate the business value of new tools to non-technical executives. And the first step to communicating clearly is to define a vocabulary to think through what machine intelligence is, how the different algorithms work, and, most importantly, what practical benefits they can provide across verticals and industries.
Shivon Zilis, a partner and founding member of Bloomberg Beta, is doing just that. She has spent the past few years focused exclusively on machine intelligence, building out a vocabulary and taxonomy to help the community understand activity in the field and communicate new developments clearly and effectively.
We interviewed Zilis to learn her views on the past, present, and future of machine intelligence. Keep reading for highlights!
Let’s start with your background. What drew you to machine intelligence?
I started off as a weird kid interested in tech but lacking local influences to get into engineering at an early age. I was also a sports nerd, and, being from Canada, my first real ambition was to create Moneyball for hockey. Unfortunately, the hockey leagues weren’t capturing data like the baseball leagues, and I definitely didn’t have the resources to pay an army to capture the data required for that kind of task. So after studying economics and philosophy at Yale, I ended up working at IBM. This was at the time when the CFO was transitioning from being a bean counter to truly setting strategic direction for organizations, so I helped with a study about that shift. After, I joined a swat team trying to figure out how IBM, as a tech company, could support the world of microfinance. The project deepened my interest in the potential of data-driven businesses, which became my focus when I moved to Bloomberg. A few years back, my former colleague Matt Turck and I realized we lacked a good framework to think about data companies – not data storing and processing companies, but those that use algorithms intelligently to derive insights and value from data. That framework became the machine intelligence landscape.
Has your degree in economics and philosophy influenced how you think about the tech industry?
You know, the landscape is a decent technical analogy for how my mind works. The combination of liberal arts and economics provided me the mental flexibility that’s needed to provide value as a non-technical person working in the tech. As an investor, I navigate back and forth between understanding the tech behind strong products and understanding the abstract market trends that govern a sector’s development. Philosophy taught me how to see how general trends unfold from first principles, and economics are the fundamentals of the business world. Most of the founders I work with are highly technical, and I have deep respect for their skills; they are the truly special ones, not me! I’ll never drill as deep as they do, but, being truly inspired by their work, we work together to build a common language where they communicate the tech and I help them understand why it matters for the rest of the world.
How do you define machine intelligence?
I consider machine intelligence to be the entire world of learning algorithms, the class of algorithms that provide more intelligence to a system as more data is added to the system. The terms “machine learning” and “artificial intelligence” don’t overlap precisely in our common lexicon, so we needed a new term. But basically, these are algorithms that create products that seem human and smart.
Besides giving a snapshot of current companies in the space, what value does the landscape provide?
One thing I noticed early on is that machine intelligence startups had a marketing problem. Pragmatic business people don’t buy magical algorithms that do everything, which was how early startups marketed their work. The landscape, in turn, provides founders with language to explain what they do to a customer or investor, and helps them ask questions like “what business problem are we solving? Are we helping with recruiting? Research? Who is going to use our tool, data scientists or marketers? etc.” Data leaders in large enterprises are also using the chart to help internal teams think about this domain and how it may shape their future tech strategy. As regards investment strategies, I’d say there are three primary benefits. First, it’s like a ledger to keep track of a rapidly changing ecosystem. Second, it helps illuminate true outliers that could become huge investment opportunities, those companies that don’t neatly fit into the established buckets. Third, and perhaps most importantly, it helps track evolution of the landscape over time, as each chart captures a state in time. We’ve noticed huge changes between the first and second versions of the chart.
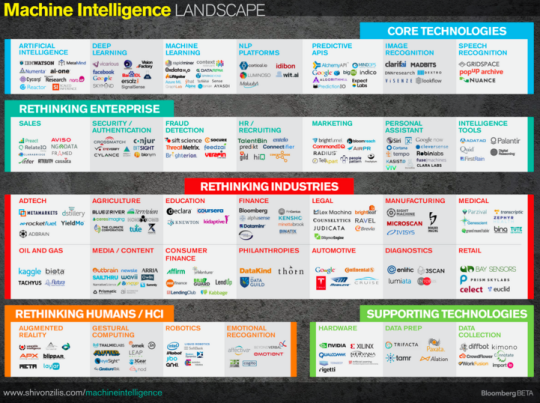
The 2014 Machine Intelligence Landscape
What changed over the past two years?
In 2014, many of the core technologies were sold as APIs. Today, there is a proliferation of companies that offer products and services built using the algorithms in the original APIs, but we’ve yet to see a parallel level of market adoption. This is a concerning trend. Alchemy API (now part of IBM) is arguably one of the most successful natural language processing startups and they struggled to break past a few million in revenue. We’re also seeing more machine learning platforms companies, but they struggle to explain to enterprises how they differ from competitors. I think the reason for this, which my partner James Cham thoughtfully pointed out, is that using machine intelligence isn’t as simple as just plugging in a technology; it requires an organizational shift in the way teams build and iterate on products, which enterprises still need help figuring out. As the market matures we will see more adoption. For a long time data science technologies had trouble selling in to the enterprise (it was too early), but the rise of data science tools reflects an underlying shift in the enterprise. Companies are starting to figure out how to productively use armies of data scientists; once teams start to expand rapidly, they open a market for tools that help with collaboration and version control tools (there was no need when it was an army of one). Finally, the most significant change occurred in the top row of the 2016 chart with the rise of the intelligent agent. What used to be packaged as a learning algorithm embedded in software to create static data output is now coming to market in form of a bot. These tools can accept multi-step instructions and negotiate on your behalf. The intelligent agent didn’t even exist as a category two years ago.
Writing about “magic wands,” SaaS tools that extract insights from data and seamlessly integrate those insights into a workflow, you mention the UI for these products should be created to “fortify the user’s knowledge rather than replace it.” Is market adoption slow because people are concerned about intelligent tools dulling their skills or automating their jobs?
That technology dulls certain skills and creates a need for new skills is not new to machine intelligence. Prior to the agricultural revolution, we could tell the difference between plant species and predict rain from the sound of a stream. Plato worried that writing, an early technology, would dull our memory. Tech companies have historically purported to save costs through automation. But I haven’t seen the same type of language in machine intelligence marketing, which tends to promise that it will help knowledge workers do their job better rather than replace them. Consider the HR job description tool Textio. People want to use this because it gives them super powers, funneling collective intelligence into their individual work. It helps to put this into perspective alongside a tool like spell check in email. Of course we want to have a machine improve our spelling! In the future, I expect people will come to use a text optimizer as naturally as they use spell check.
What virtual agents? Daniel Tunkelang wrote an interesting Medium post recently describing how we “trust computers to do things for us, but not as us.” Will the market require a cognitive shift to adopt virtual agents?
It depends on the product category. We have a summarization product in our portfolio (like the prototype you built at Fast Forward Labs) that tells you what you need to know about longer articles you have to read. Assistants like this have a low barrier to adoption. It’s the same with Textio, where users literally just pop in text they’ve written and get analytic feedback. Assistants that engage with others on your behalf have to deal nuances you may or may not even know exist. Using tools like a scheduling assistant can require upfront energy and investment, just like a human relationship! Sure, you can get married, share resources, and basically live separate lives, or you can invest the time required to build closeness and appreciate the nuances of another person.
What do you predict will happen to the machine intelligence market over the next year?
First, I think companies will make progress building great data science teams, and integrate data science more tightly into other business units. I think we’ll see a lot of investment in machine intelligence companies focused on healthcare, and a bunch of logistic companies that can support self-driving cars developed by larger players. I personally would love to see more applications in education, as there could be so much upside for society at large. It’s hard to sell into the education vertical, and really requires a brave individual to take on the task. We’re also really excited about companies like Gridspace that are capturing and processing audio data. There is so much data lost from live meetings or calls that could provide incredible insights. Finally, I’m slightly concerned all the bots being built could rapidly lead to overload and disenchantment. As with the mobile app explosion, the barrier to building a semi-functional bot is not very high, and that could lead to a lot of annoying bots pinging us needlessly. The risk lies in the gap between the hyperbolic expectations generated by the press and the rather banal reality of most products’ performance.
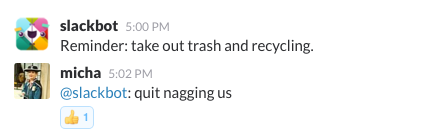
Fun times with the Fast Forward Labs slackbot.
What advice would you give to aspiring entrepreneurs?
A founder needs to be obsessed with a given problem to be successful. She needs to believe so deeply in the mission that she is resilient through criticisms, not winning a funding round, or not immediately winning customers. There are so many businesses out there, but those that are well backed are the outliers that are ready to devote 10 years of their life to one problem, ready to wake up every morning and passionately embrace their work. Today, there are many lucrative options for people with machine learning talents, as large companies are building their teams. We single out those few individuals who show us they’re willing to remake the world to solve their problem.
- Kathryn